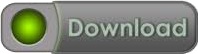
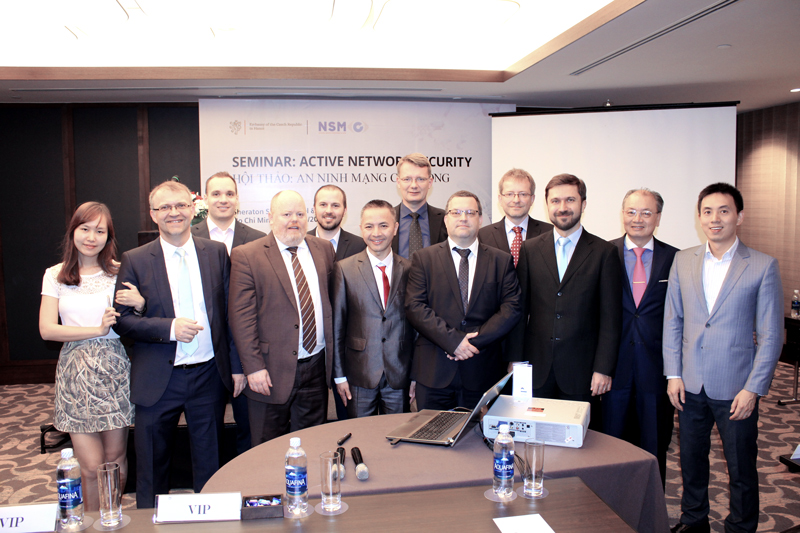
Ide & Pustejovsky (2010) suggested a list of best practices for language technology metadata, focusing heavily on the work of the OLAC and European Languages Resource Association (ELRA).
Babelnet vs framenet iso#
Bird & Simons (2003a) and Ide, Romary, & de la Clergerie (2004) proposed sets of best practices for linguistic annotations, while Simons, Bird, & Spanne (2008) offered a more recent set of recommendations that specifically suggested language codes from ISO 639-31 be used in metadata. A brief history of the topic of linguistic annotation can be found in Palmer & Xue (2013). Linked Linguistic Open Data (LLOD) is heavily dependent on metadata, and any consideration thereof would require an examination of its standards. SemEval is an ongoing evaluation project which is used as a baseline to assess various WSD methods, including many which will be examined in this paper. Generalists might find sufficient the survey from Navigli (2009), or the chapters covering WSD in either Jurafsky & Martin (2009) or Manning & Schutze (1999). Kwong (2013) offers slightly more recent coverage, along with predictions as to how WSD methods will evolve in the near future. The most complete treatment of the subject of WSD is arguably Agirre & Edmonds (2007), which presents a detailed definition of the problem, along with a history thereof, and numerous algorithms which are used in practice. This paper will examine several systems that purport to disambiguate words by using Linked Data, and some of the models these systems use to ensure interoperability. Linked Data technologies (Berners-Lee, 2006), however, allow us to utilize existing ontologies and lexica, which can then be exploited to improve the automatic semantic understanding of the word.
Babelnet vs framenet manual#
Furthermore, these methods have been heavily dependent on the manual creation of knowledge sources (Edmonds, 2000), which are expensive to create and subject to change, thus creating what is termed a knowledge acquisition bottleneck (Gale, Church, & Yarowsky, 1992). ), how is an information retrieval system to understand which sense of the word is intended? There exist tried-and-tested methods, such as just using the most predominant sense of the word (McCarthy, Koeling, Weeds, & Carroll, 2004) or looking at the words next to the query term to determine the statistically most likely meaning (Jurafsky & Martin, 2009 Manning & Schutze, 1999) but these methods often produce less-than-satisfactory results (Navigli, 2009). If someone makes a query for a polysemous word (e.g., "plant," "bass," "mercury," etc. Word Sense Disambiguation (WSD) is referred to as an "Al-complete" problem (Mallery, 1998), i.e., a task that is relatively easy for people, but considerably more difficult for machines.
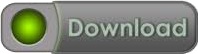